How to Determine Which Research Design to Use
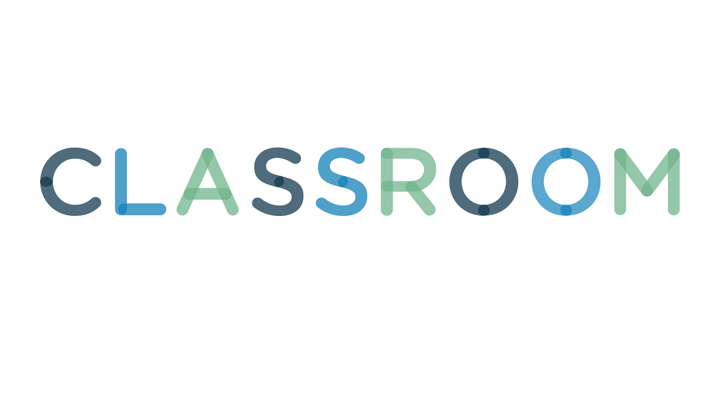
Content and purpose of the study determine the best design for any research project. The whole process starts with a defined problem, or the question that the study aims to answer, and ends up with the complete study design which prescribes exactly what and how is measured and how it is going to be analyzed.
All types of research require a careful design in the sense of planning, while the term “research design” has also a specific meaning applying to quantitative, and particularly experimental and quasi-experimental studies.
- General Research Design
- Define the problem
- Review literature and previous research results
- Define the population
- Define the variables in operational terms
- Formulate the hypothesis
- Choose the broad approach : qualitative
- Quantitative Design
- Define your main goal
- Conduct
- Randomly assign subjects
- Start
- Scale back such a fully developed design
1 General Research Design
2 Define the problem
Define the problem. This is by far the most important step in the process of designing a research project. Defining the problem starts with picking a theme, or a topic area, and proceeds to ask a specific question.
3 Review literature and previous research results
Review literature and previous research results. This will provide the theoretical framework as well as guide the thinking. Gaps in the current knowledge, questions remaining unanswered as well as research approached and classic studies can all be found in existing data. In the business context, review existing market research reports and check if the problem can be solved by secondary-source research, almost always cheaper and faster than commissioning a new study.
4 Define the population
Define the population. Many psychological studies aim to uncover general truths about how people function. Marketing and sociology usually define their populations more specifically, by narrowing it down to users of a product, people in one city or town, or a certain demographic group.
5 Define the variables in operational terms
Define the variables in operational terms. Opertionalization means knowing not only what phenomena you are going to study, but also how they are going to be measured. Some variables are pretty self explanatory (sex, age) but others need to be precisely defined. Even seemingly straight-forward variables might need consideration: for example attending day-care might be defined in simple yes-no terms, measured as a number of hours per week on average, or a number of months before the age of 4 years old.
6 Formulate the hypothesis
Formulate the hypothesis. The hypothesis defines the rest of the research process. It is the proposition that you are going to test in your research and needs to include specific predictions as to the relationship between the variables. The only situation in which there is no hypothesis is a purely exploratory, descriptive study.
7 Choose the broad approach : qualitative
Choose the broad approach: qualitative, quantitative or mixed. In the academic, this choice is as much a matter of philosophy and principles as suitability. In business and other applied research situations, qualitative approach is usually chosen to explore reasons and ways of thinking, while quantitative approaches are used to gather data that can be generalized or to conduct cause-and-effect investigations.
8 Quantitative Design
9 Define your main goal
Define your main goal, keeping in mind the hypothesis. If it's description and generalization, choose a survey methodology. If it's investigating a cause-effect relationship, consider an experimental or quasi-experimental study.
10 Conduct
Conduct an experimental or a quasi-experimental design if it's possible to have a control group or multiple waves of measurements. This implies that you can control the independent variable, deciding whether and when it is applied. Such application is called “treatment.” If you cannot control the independent variable for practical or ethical reasons, conduct a correlation study.
11 Randomly assign subjects
Plan a true experiment if you can randomly assign subjects to experimental groups, i.e. every person has as much chance of ending up in the treatment group as in the control group. If you have to use pre-existing or otherwise non-equivalent groups, conduct a quasi-experiment.
12 Start
Start with the simplest design for the experiment or quasi-experiment that demonstrates the hypothesis. Then add all the extra variables, control groups, measurement methods and measurement waves you can think of.
13 Scale back such a fully developed design
Scale back such a fully developed design, which is likely to be impossible to realize practically. When deciding which factors, measures or groups to remove, always consider possible confounding variables and explanations alternative to your hypothesis.
- Don't overstretch the resources. Leave yourself a margin of money and time for the unexpected. Things will go wrong and the larger the study the more likely it is.
- Plan the data analysis when designing the study. If you don't know what main analyzes you are going to conduct, you are likely to find out that an important measurement is missing. This is especially crucial in large-scale surveys.
- Consider a pilot study, especially if you are using a new instrument (a questionnaire, a scale) or a new way of introducing the stimuli.
- Consider the ethical dimension. If the experiment involves deception, build in careful debriefing. If the study evaluates medical, educational or social interventions such as drugs, remedial programs or therapies, consider how a random (or non-random) assignment to groups might affect the real-life situation of the subjects.
- A validity of an experimental study depends on accounting for possible alternative explanations. Taking into account possible confounding variables is crucial. If possible, choose a factorial design and incorporate them into the study.
- Correlation studies don't allow for causal inference but only for measuring of associations. Good theory and certain types of analysis might make possible causal inference stronger in such cases.