What Does Normality Mean in Statistics?
26 SEP 2017
CLASS
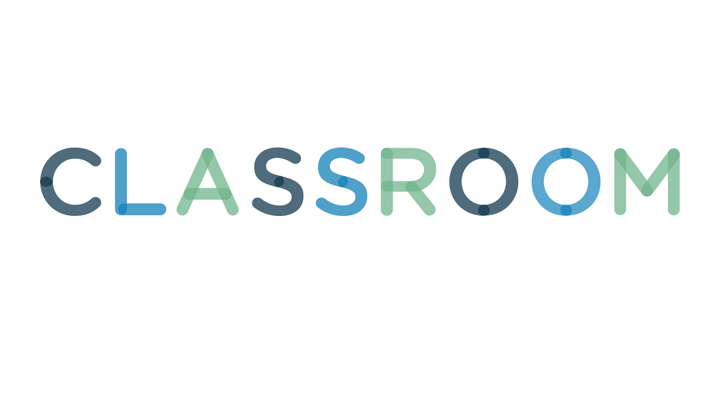
Normality is a key concept of statistics that stems from the concept of the normal distribution, or “bell curve.” Data that possess normality are ever-present in nature, which is certainly helpful to scientists and other researchers, as normality allows us to perform many types of statistical analyses that we could not perform without it. Students should remember to check a dataset for normality before performing an analysis that relies on normally distributed data.
1 Normal Distribution
The term “normality” actually derives from a more basic statistical concept: the normal distribution. The normal distribution describes the “shape” of a population as being in that of a bell curve. That is, when you plot the statistics along a horizontal axis for that particular variable -- time, for example -- with the vertical axis representing the probability of observing that value on the horizontal axis, a dataset with a normal distribution will have a shape like a symmetrical mountain: high in the middle and gradually sloping down to the left and right. Data that follow such a distribution are said to possess normality.
2 Examples
Normality exists throughout nature. Many variables, from the amount of ketchup the average person squeezes onto his hotdog to the average lifespan of a laptop computer, possess normality. What this means is that these variables are likely to fall around the middle value, known as the mean or median, of the normal distribution, but are also as equally likely to fall to the left or right of that middle value. For example, the normal distribution can describe the time of human gestation. Though we have a habit of saying that pregnancy lasts nine months, it doesn’t always last exactly nine months. In fact, the length of one woman’s pregnancy likely differs by several days from that of another, with some shorter and others longer.
3 Why it’s useful
Normality is an important concept in statistics, and not just because its definition allows us to know the distribution of the data. According to statisticians Robert Witte and John Witte, authors of the textbook “Statistics,” many advanced statistical theories rely on the observed data possessing normality. For example, a t-test, which allows statisticians and other researchers to check the probability as to whether two variables come from different distributions, requires that the data you’re working with possess normality. If not, you cannot apply the t-test, which makes statistical analysis much more difficult.
4 Checking for Normality
Understanding normality is one thing; determining whether a dataset possesses normality is another. Statisticians often go to great lengths to check if their data possess normality. Using the traits of the normal distribution, you can check a dataset for normality in many different ways. The easiest ways include plotting the data and observing whether the data appears to fit a bell-curve shape or checking the skew of a dataset, which can tell a researcher whether the data is symmetrical -- normality requires symmetry. On the harder side are rigorous statistical tests that can tell statisticians within a certain degree of certainty whether a dataset possesses normality. No matter which way you use, ensuring normality before performing an analysis can safeguard the appropriateness of your methods.
- 1 Statistics; Robert Witte, John Witte
- 2 The University of New Mexico: Testing Assumptions: Normality and Equal Variances