How to Collect Data From a Science Project
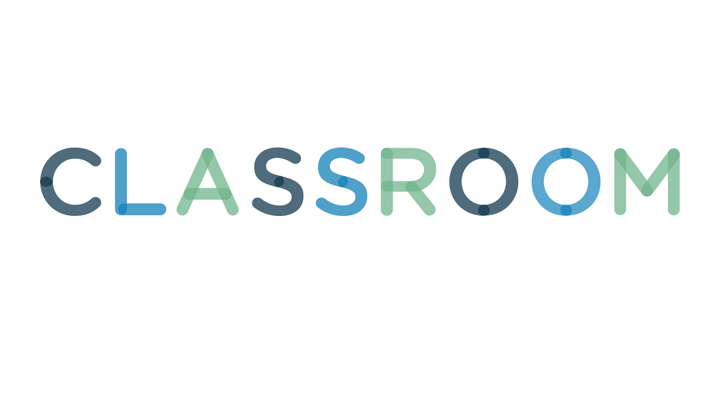
Proper and efficient data collection from a science project that is short-term or long-term has multiple benefits: It makes data analysis much easier, it makes tracking oddities much easier and it maximizes the information that can be extracted from the data. Data collection can be done quantitatively -- that is, using numerical measurements such as height and weight. Data collection can also be done qualitatively, or using descriptions such as "light-colored" or "dark-colored." Lastly, having an organized system of labeling experiments, treatment groups and samples will make data analysis much more fruitful.
1 Measure It
Quantitative measurements record changes in the experiment in numerical form. Numbers can be analyzed by mathematics, meaning they can give very accurate degrees of change. Measuring the speed of a solar-powered car, for example, yields a good idea about the strength of different batteries that are being tested. Measuring how much solid precipitate forms in a chemical reaction provides information about how readily the reactants interact with each other. Quantitative measures allow you to calculate the inaccuracy of repeated measurements or repeated experiments -- known as the percent error. Quantitative measures also allow for statistical analysis that tells you whether the results were derived from more than just random chance.
2 Describe It
Qualitative measures partition results into descriptive categories. For example, a chemical reaction produces a liquid that can be described as clear, pink or red. Qualitative measures can be done more easily than quantitative measures but provide less information. A method of data collection called semi-quantitative measurements, however, are qualitative measures that are repeated multiple times. For example, even though "clear," "pink" and "red" are not numbers, three repeated experiments might all yield approximately 10/100 (10 percent) clear and 90/100 (90 percent) red liquid -- suggesting that the experiment reliably yields 90 percent red liquid every time. Semi-quantitative analysis quantifies a qualitative measure, allowing the researcher to apply statistical tests to determine if the effect is real or just due to random chance.
3 Have Triplicates and Repeats
Each condition being tested should have triplicates, meaning each treatment group should have at least three samples. For example, if you're testing the effect of sunlight on the growth of potatoes, the sunlight-treated group and the kept-in-the-dark group should each have more than one potato. This prevents unforeseen problems. For instance, if there is only one potato and it happens to be rotten or accidentally eaten by your pet dog, your several-week-long experiment will be destroyed. Furthermore, if you have two, three or more potatoes in each treatment group, you don’t have to worry about natural differences in the growth rate of individual potatoes skewing the effect of sunlight. Consider that your brothers and sisters do not all have the exact same height, though they are from the same family. Lastly, repeat the experiment at least twice to see if you get the same results, and organize your data in the format of a data table.
4 Keep an Organized Notebook
Real-world data is messy, whether it’s from a high school science project or a university laboratory. Thus, keeping an organized notebook that records what happens each day or each time data is collected helps make the data analysis easier. It is helpful to have a systematic labeling system for each experiment, each treatment group and each sample. For example, "E3-G4-S3" would tell you that this sample is from experiment 3 (E), treatment group 4 (G), and is sample No. 3 (S). Many Nobel Prizes have been given to what started out as an oddity that some researcher refused to ignore. Systematic labeling also makes it easier to refer to other related experiments in your notebook that were done at different times: “Experiments E1 and E5 had similar results.” It also helps to identify experiments that might have been accidentally ruined for unknown reasons, especially if a group of data points from one experiment behaves differently than all the other experimental repeats.